Clinical Decision Transformer
: Intended Treatment Recommendation through Goal Prompting
Code: soon be available
Summary
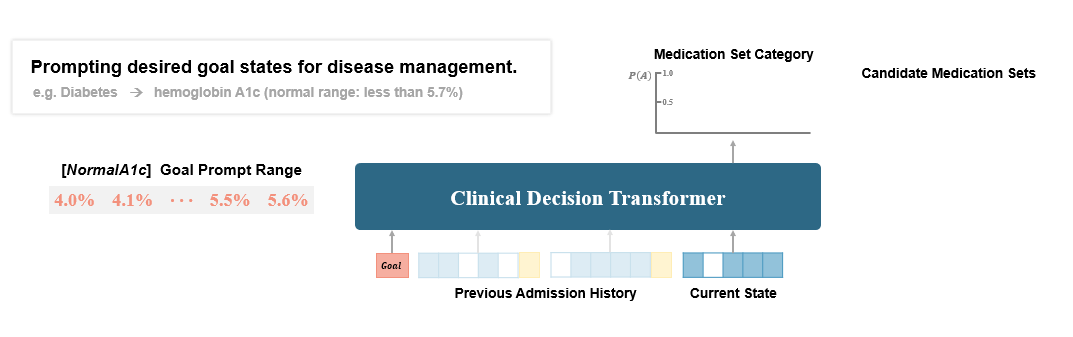
-
We extracted a diabetes dataset from an electronic health record (EHR) system and used it to train two models; the proposed Clinical Decision Transformer and Counterfactual Recurrent Network [1] as an evaluation model for estimating recommendation effects.
-
The evaluation model estimates a counterfactual clinical state of the very next admission, given previous admission history and currently recommended medications by the Clinical Decision Transformer.
-
We find that recommended medications by the Clinical Decision Transformer shift patients' clinical states (e.g. hemoglobin A1c) in the intended directions (e.g. the normal range of hemoglobin A1c: 4–5.6% [2]) compared to those by factual prescriptions and behavior cloning.
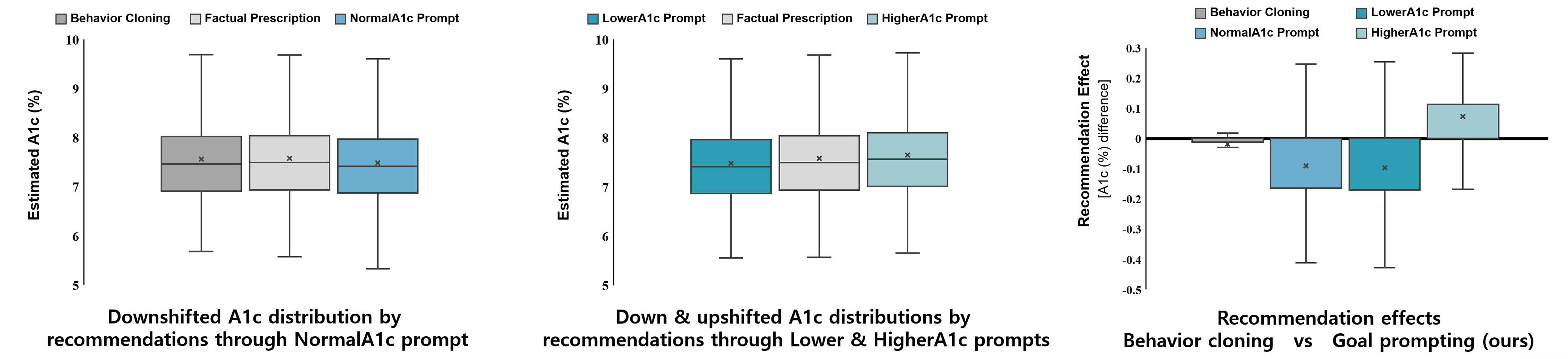
Contextual Embedding
-
It is necessary to contextualize inter-admission and intra-admission information to fully utilize large-scale EHR data characterized by heterogeneity and high missing rates (for laboratory test columns in our dataset; mean 44.39%, median 22.37%).
-
In a t-SNE analysis, we observe that the output embeddings for each admission point are clustered with respect to prescriptions, while the input admissions are entangled before contextualized.
-
In this study, the cold start corresponds to the case where the input admission length is one without previous admission history.
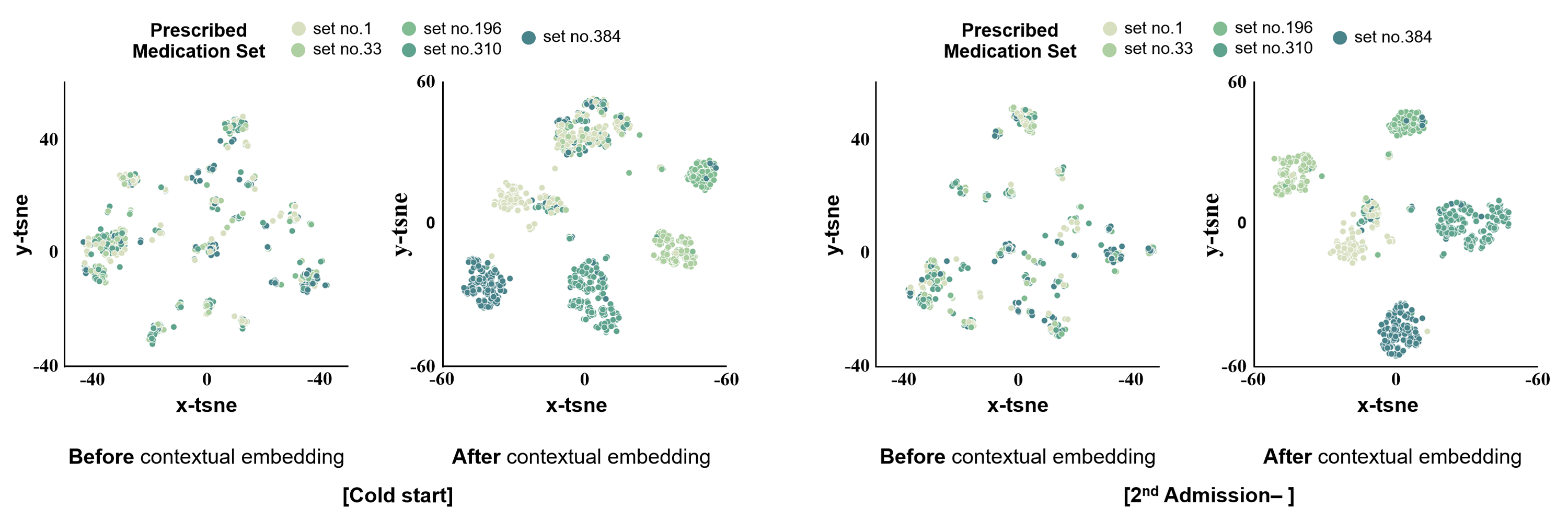
Attention Pattern
-
We visualized representative cases of attention patterns to analyze how the Clinical Decision Transformer recommends medications and contextually embeds sequential EHR data.
-
We find that the model tends to utilize recent inter-admission information to contextualize the clinical states of the current admission, while when previous information is insufficient, intra-admission contextualization becomes more active.
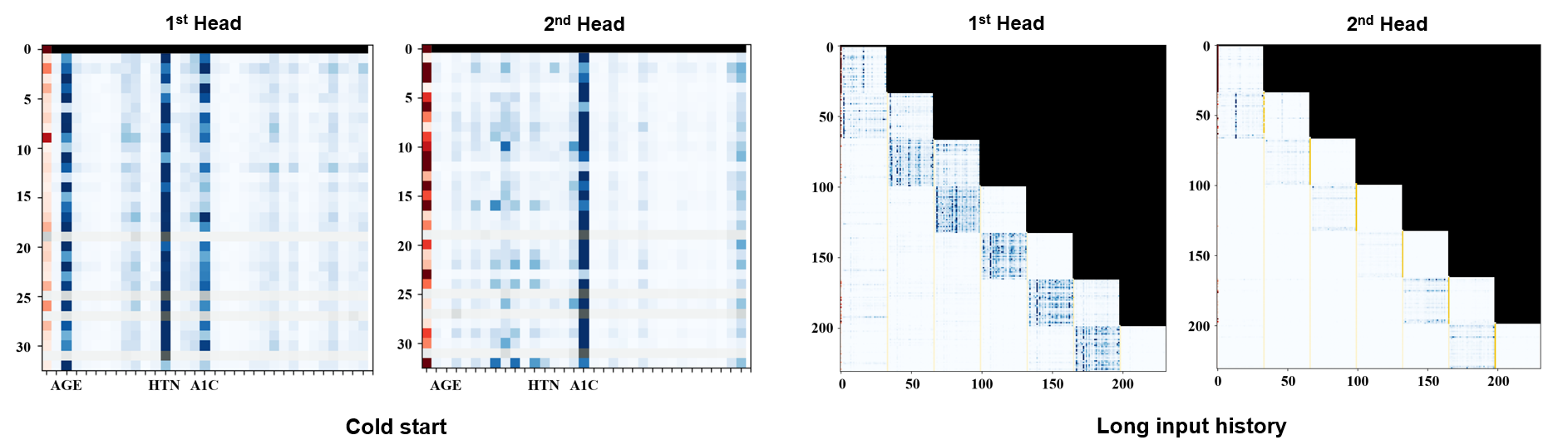
Abstract
In this paper, we propose Clinical Decision Transformer (CDT), a recommender system that generates a sequence of medications to reach a desired range of clinical states given as goal prompts. For this, we conducted goal-conditioned sequencing, which generated a subsequence of treatment history with prepended future goal state, and trained a GPT architecture to model sequential medications required to reach that goal state. In an experiment, we extracted a diabetes dataset from an EHR system, which contained treatment histories of 4788 patients. We observed that the CDT achieved the intended treatment effect according to goal prompt ranges (e.g., NormalA1c, LowerA1c, and HigherA1c), contrary to the case with behavior cloning. To the best of our knowledge, this is the first study to explore clinical recommendations from the perspective of goal prompting.
Keywords: Clinical Recommender System, Clinical Decision Support Systems, Human-AI Interface, Electronic Health Records, Causal Inference
References
[1] Bica, I., Alaa, A. M., Jordon, J., and van der Schaar, M.
Estimating counterfactual treatment outcomes over time
through adversarially balanced representations. arXiv
preprint arXiv:2002.04083, 2020
[2] American Diabetes Association. Understanding A1c.
* The data extraction process from the EHR system was approved by an official review committee.